The fastest-growing data source in the world, the healthcare industry now accounts for 30% of global data. That’s thousands of exabytes of data. And medical images comprise from 80% to 90% of healthcare data — an insurmountable amount for any human to process.
Technology, and namely machine learning, is stepping up to help healthcare professionals cope with the task at hand. ML-supported medical image analysis comes with the promise to automate time-consuming processing workflows, improve diagnostics accuracy, and ultimately drive better patient outcomes. But even though AI-based algorithms can outperform doctors in a variety of applications, patients have their doubts as to the accuracy of these systems.
To understand how reliable medical image analysis software is, first let’s have a look at what’s under the hood.
Medical image processing pipeline
As mentioned earlier, at the heart of medical image processing lies machine learning. With the ability to crunch through great amounts of data in a fraction of time, deep learning algorithms help physicians analyze and interpret medical imaging, catching even the slightest details.
The supported medical imaging modalities include:
- Computed tomography (CT) scans
- Magnetic resonance imaging (MRI)
- X-ray imaging
- Positron emission tomography (PET) scans
- Ultrasound imaging
- Single-photon emission computerized tomography (SPECT) scans
- Radiographic imaging
As shown on the diagram below, core stages include preprocessing, segmentation, extraction, and classification.
Source: Computer-Aided Diagnosis
Pre-processing. At this stage, the raw image is processed to enhance quality and ensure further computation process goes faster. Pre-processing activities include removal of noise and background artifacts, leveling or increasing the contrast.
Segmentation. One of the core tasks in medical image analysis, segmentation means partitioning the image into sets of pixels i.e., segments in order to locate objects and boundaries. These segments are the regions with similar properties like color, texture, brightness, and contrast. High variability within an image as well as multiple image modalities make this task a challenging one.
Feature extraction. After segmentation, regions of interest (ROI) are further analyzed for specific characteristics like gray levels, texture, patterns, distortions.
Classification. Finally, a classification algorithm is used to assess every ROI for being true positive, false positive, true negative, and false negative. If the detected structure reaches the threshold level, the system automatically flags it as abnormal.
Case in point
An automated pneumonia diagnosis tool, a part of a comprehensive telehealth platform, was designed to help identify signs of pneumonia using machine learning techniques. For that, the tool leverages the convolutional neural network Inception v3 developed by Google Research Team. The developed model was further trained using a curated data set of lung images.
The resulting algorithm uses binary identification — if it identifies 80% of lungs as unaffected, it will mark the lungs as healthy. For anything less than 80%, the model assumes the lungs might be affected and require expert medical attention. In addition to significantly reducing human errors, the solution can alleviate the burden of healthcare professionals.
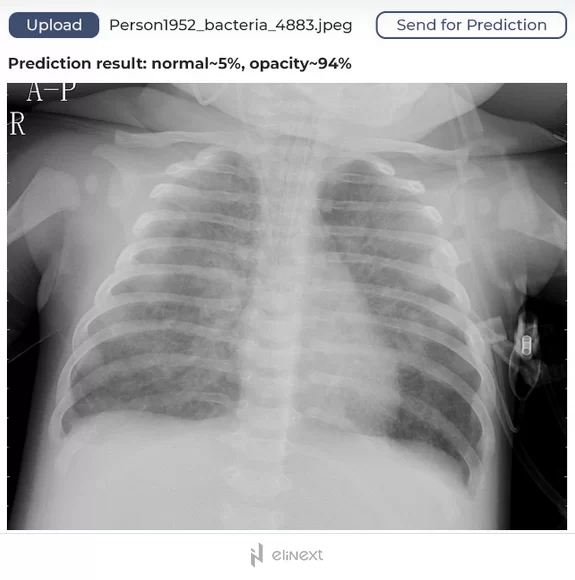
The advancements in deep learning have changed the dynamics of the medical image solutions adoption. By 2028, the global AI-powered medical imaging solutions market is expected to amass USD 1.5 billion.
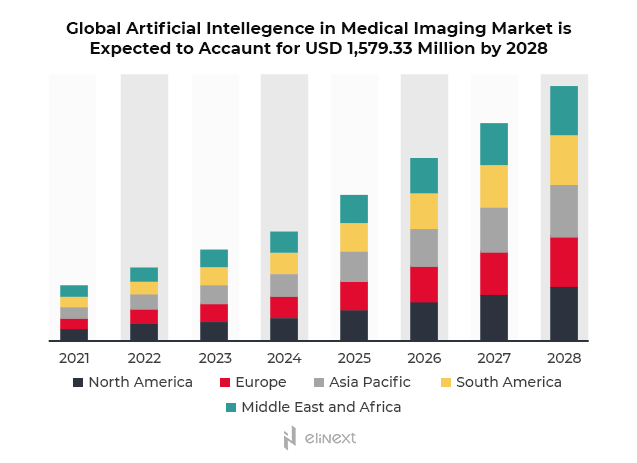
From detecting breast and skin cancer to identifying cardiac pathologies, automated medical image analysis enables medical professionals to arrive at an accurate diagnosis faster, which significantly improves survival rates. With so much at stake, it’s only natural to wonder how reliable and accurate these algorithms are.
Is AI-based medical image analysis on par with healthcare professionals?
A team of researchers carried out the first systematic review with the purpose to compare the performance of AI-powered medical image solutions with that of healthcare professionals. The results are published in the Lancet Digital Health journal.
The scientists focused on two metrics — sensitivity and specificity. Sensitivity is the ability of the diagnostic tool to generate a positive result for patients with a disease (true positive rate). Specificity measures the tool’s ability to generate a negative result for patients without a disease (true negative rate).
The meta-analysis revealed that the performance of deep learning models and medical experts is equivalent. DL models showed a pooled sensitivity of 87% and a pooled specificity of 92.5%, while healthcare professionals demonstrated 86.4% and 90.5%, respectively.
Are there any limitations?
Any machine learning model needs training, that’s why the ML-powered medical image analysis system is just as good as training data sets. Any biases found in the training data will be translated and amplified in the ML model. These biases can be introduced through variables like gender, race, age, and even insurance — those who lack proper health insurance have limited access to medical care and can be underrepresented in data samples, which results in skewed models.
Fair and unbiased data sets are must to ensure the accurate performance of an AI-enabled medical image analysis solution. One of the ways is to identify potentially discriminatory behavior already during the data processing and preparation stage. Other activities may include:
- Including key variables into the ML algorithm
- Testing ML models against diverse socioeconomic conditions
- Continuous monitoring and verifying the validity of the ML model output
Who calls the shots — a doctor or a system?
AI-based healthcare solutions are becoming an integral part of the care delivery ecosystem, and rightfully so. Automated medical image solutions provide reliable clinical decision support, accelerate time to diagnostics, and enable high-quality patient care.
However, patients are more comfortable if a physician remains in charge of the ultimate decision regarding the course of treatment. In one study, the respondents admitted they would be as likely to let the algorithm analyze their body scans for skin cancer and then make recommendations to a physician as they would be to rely on the treatment provided by a doctor from start to finish.
Key takeaways
Artificial intelligence is rapidly gaining ground across a variety of industries, and medical diagnostics is no exception. From patient apps for body tan identification to medical practice systems enhanced with medical image processing capabilities, AI can augment healthcare in a number of meaningful ways, enhancing productivity and decreasing costs due to reduced errors and misdiagnosis.
With today’s level of tech maturity and readiness of patients to embrace AI-based medical services, automated medical image analysis software remains a tool, although powerful, in the hands of experienced healthcare professionals who verify the result and adjust the course of treatment. But armed with these AI-powered capabilities, physicians can reach populations on a much larger scale, significantly improving outcomes and enhancing the quality of patient care — the ultimate goal for healthcare.